Motion control engineer Fabian Rudnick shares insights into PI's development of learning-based motion control for the future.
“What drove us was achieving motion performance others deemed impossible.”
Technology Leadership Interview: The Future of Learning Based Motion Control | PI
Technology Leadership Interview: The Future of Learning Based Motion Control. Video Credit: PI (Physik Instrumente) LP
The Driving Factor in the Development
The primary motivation for the PI’s team was achieving motion performance considered impossible by others. This pertains to the Bode sensitivity integral, which implies that enhancing one frequency range necessitates redistributing elements across the frequency spectrum. There is always a trade-off.
PI's new motion control technology circumvents this fundamental control theory limitation, significantly enhancing performance.
Circumventing Servo Bandwidth Limitations
This technology empowers PI's customers to achieve superior disturbance rejection. Traditional feedback and feedforward servo systems are constrained by servo bandwidth, limiting the frequency of disturbances they can handle.
With this innovation, compensation for disturbances extends well beyond this limit. Consequently, customers can expect improved standstill precision, superior disturbance rejection, and overall enhanced motion performance across various applications.
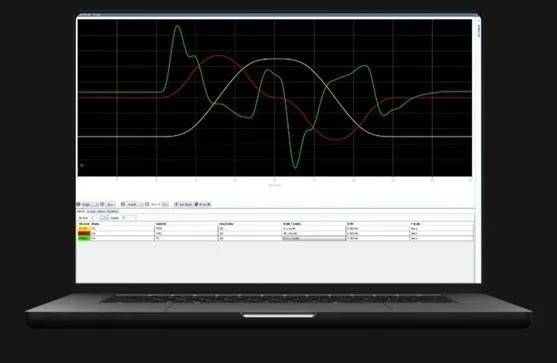
Image Credit: PI (Physik Instrumente) LP
New Algorithm Extends Lifetime of Existing Mechanical Design
The development of these algorithms greatly benefited from extensive and enduring relationships with PI's customers. For instance, one customer aimed to overhaul a machine due to an inability to meet specifications for a new iteration with existing hardware.
Implementing the new algorithm improved motion performance on the old machinery, surpassing the new specifications. The expenses of redesigning mechanics were saved by simply applying the algorithm.
Ongoing Development – Reduction of Following Errors by 10X
It is important to note that this development is still in progress. PI is continuously refining this new technology to enhance its robustness and user-friendliness in customers' applications. Ensuring stability with this new technology presents a significant challenge when compared to the traditional linear time-invariant systems.
During the initial trial of this new algorithm at a customer site, simply activating this feature resulted in an immediate 10-fold reduction in subsequent errors. Witnessing the error diminish while observing the machine and software oscilloscope was incredibly exciting.
Learning-Based Motion Control Algorithms in Brief
Control algorithms relying on feedback and feedforward methods can achieve impressive performance but come with inherent limitations.
- Preventative disturbance compensation: Machine learning control algorithms for precision motion systems enable proactive compensation for disturbances and intelligent real-time optimization of feedback and feedforward controls.
- Multiple learning modes for higher performance: Learning from various past execution modes minimizes move-and-settle time, reduces dynamic following errors, and maximizes stability across a wide range of operations.
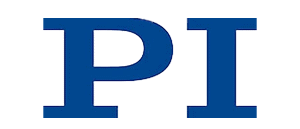
This information has been sourced, reviewed and adapted from materials provided by PI (Physik Instrumente) LP.
For more information on this source, please visit PI (Physik Instrumente) LP.