In this interview, Prof. Dr. Sergei Kalinin discusses how machine learning is allowing for automation in microscopy and the future of AI in research.
Can you tell us how you first became involved in microscopy and how you use it in the context of electromechanical measurements?
I believe my first involvement with scanning microscopy occurred when I was a visiting undergraduate student at POSTECH in South Korea in the mid-1990s. At that time, I had the opportunity to learn about atomic force microscopy (AFM).
Very few research groups were working on it then, which made it seem suitably mysterious and intriguing. I decided to travel to South Korea for six months to learn everything I could about AFM.
As it turned out, the laboratory there was not fully equipped to conduct AFM research. However, there was a well-stocked library, and I can honestly say that I read nearly everything that had been published in the field of AFM and scanning tunneling microscopy (STM) from their inception up until the mid-1990s.
With that foundation, I began searching for a graduate position in the United States and joined Professor Dawn Bonnell's group at the University of Pennsylvania. The group specialized in STM and AFM, and that is where I began working with AFM for my doctoral research.
From there, I moved on to Oak Ridge National Laboratory to continue my work with AFM and STM, and that has essentially defined the trajectory of my career.
As for electromechanical measurements, that journey began during my time at the University of Pennsylvania. My advisor introduced me to an interesting technique called piezoresponse force microscopy. She asked me to explore the method and investigate what insights it could offer, particularly regarding ferroelectric materials. That was essentially the starting point for my work in electromechanics.
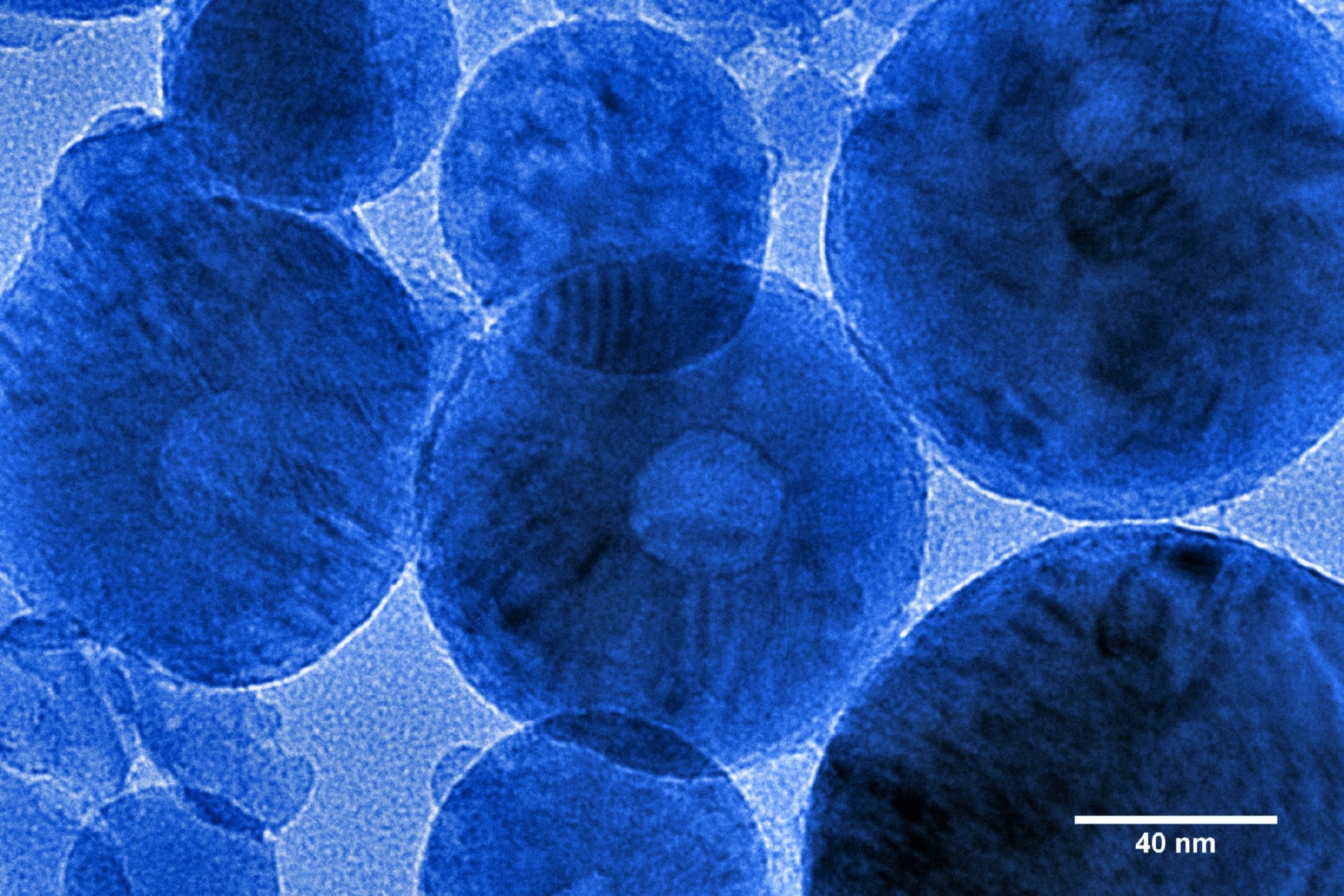
Image Credit: Georgy Shafeev/Shutterstock.com
What materials do you focus on, and why are you particularly interested in studying them?
My primary focus is more on microscopy than on any specific class of materials. However, as one can imagine, microscopy and materials research often go hand in hand.
In terms of material systems, I am particularly interested in those that exhibit electromechanical responses. Ferroelectric materials are the first class of materials that fall into this category. They exhibit relatively small electromechanical responses, typically on the order of several picometers per volt or several tens of picometers per volt.
The great advantage of using a scanning probe microscope is that it allows us to do two key things. First, we can not only image these materials and obtain high-resolution images of ferroelectric domains, but we can also conduct physical experiments by switching the polarization up and down.
Interestingly, over the past 20 years, this capability of scanning probe microscopy, to both image and manipulate polarization, enabled us to build the first artificial intelligence-enabled automated scientist.
The second important aspect of piezoresponse force microscopy is that its signal formation mechanism is intrinsically quantitative. While most scanning probe microscopy modalities can acquire beautiful images, it is often very difficult to translate those images into numerical data that characterizes the performance of specific materials.
This challenge arises from a fundamental physical limitation: in many SPM techniques, the signal depends on the contact area between the probe and the sample, and we generally cannot determine or calibrate that area accurately.
However, in piezoresponse force microscopy, the signal is independent of the contact area, making it, at least in principle, a quantitative technique. This allows us to explore material properties at the nanometer scale with real numerical insight.
Much of the work I have done over the past two decades, including the development of multimodal SPM techniques and machine learning-enabled SPM, has been rooted in the ability to obtain quantitative data.
The third area of my interest extends beyond traditional ferroelectric materials. While working at Oak Ridge National Laboratory, my colleagues and I discovered that strong electromechanical responses aren’t limited to ferroelectrics. Ionic materials, like those used in batteries and fuel cells, also exhibit significant electromechanical behavior.
In these cases, the technique can’t be described as piezoresponse force microscopy, since the signal doesn’t stem from piezoelectricity. Instead, we refer to it as electrochemical strain microscopy. Despite the different origins, the core principle behind the measurement remains consistent.
By studying both ferroelectric materials and ionically conductive solids, we touch on many of the most active frontiers in materials science and condensed matter physics—from energy storage and conversion to memory devices and beyond. It’s a genuinely exciting and fast-evolving field to be part of.
You are pioneering the use of AI in automated experimentation, which may be an unfamiliar term for many of our readers. Could you explain what automated experimentation is and why it matters to scientists?
There are several ways to think about automation. From one perspective, consider a situation where we have a large sample, but the scanning range of an atomic force microscope (AFM) is limited. In such a case, we might use the AFM to collect a dense grid of images to map the entire surface of the sample. One could argue that this is a basic form of automated experimentation.
Upon closer reflection, there seems to be something limited about that definition. Over time, and by that, I mean over the past six years or so, during which my group has worked on automated microscopy, we have come to understand that the core idea of automated experimentation should focus on replicating the operations and decisions typically made by a human operator.
To illustrate this, let us consider a typical day in the life of a microscopist. The scientist takes a sample, places it in the microscope, and proceeds through a series of actions: tuning the instrument, locating regions of interest, zooming in and out, possibly performing spectroscopic measurements, and so on.
Throughout the day, there is a continuous process of dialogue and decision-making. The human operator observes the data streaming from the instrument, interprets and contextualizes it using prior knowledge, and then decides on the next course of action, which is implemented in real time.
From this viewpoint, automated experimentation refers to a process in which a machine learning algorithm—or, more broadly, an artificial intelligence (AI) agent—takes over some or all of the decision-making that would typically be carried out by a human.
Instead of the human deciding every next step, the AI agent interprets incoming data from the microscope, makes informed decisions based on that data, and executes those decisions through the instrument.
At least initially, the quality of decisions made by the AI may not match that of a skilled human operator. However, the AI agent has key advantages: it can make decisions much faster and can operate continuously without fatigue.
In our framework, automated experimentation is defined as a scenario in which decision-making is either augmented or fully managed by an AI agent, fundamentally changing how scientific experiments are conducted.
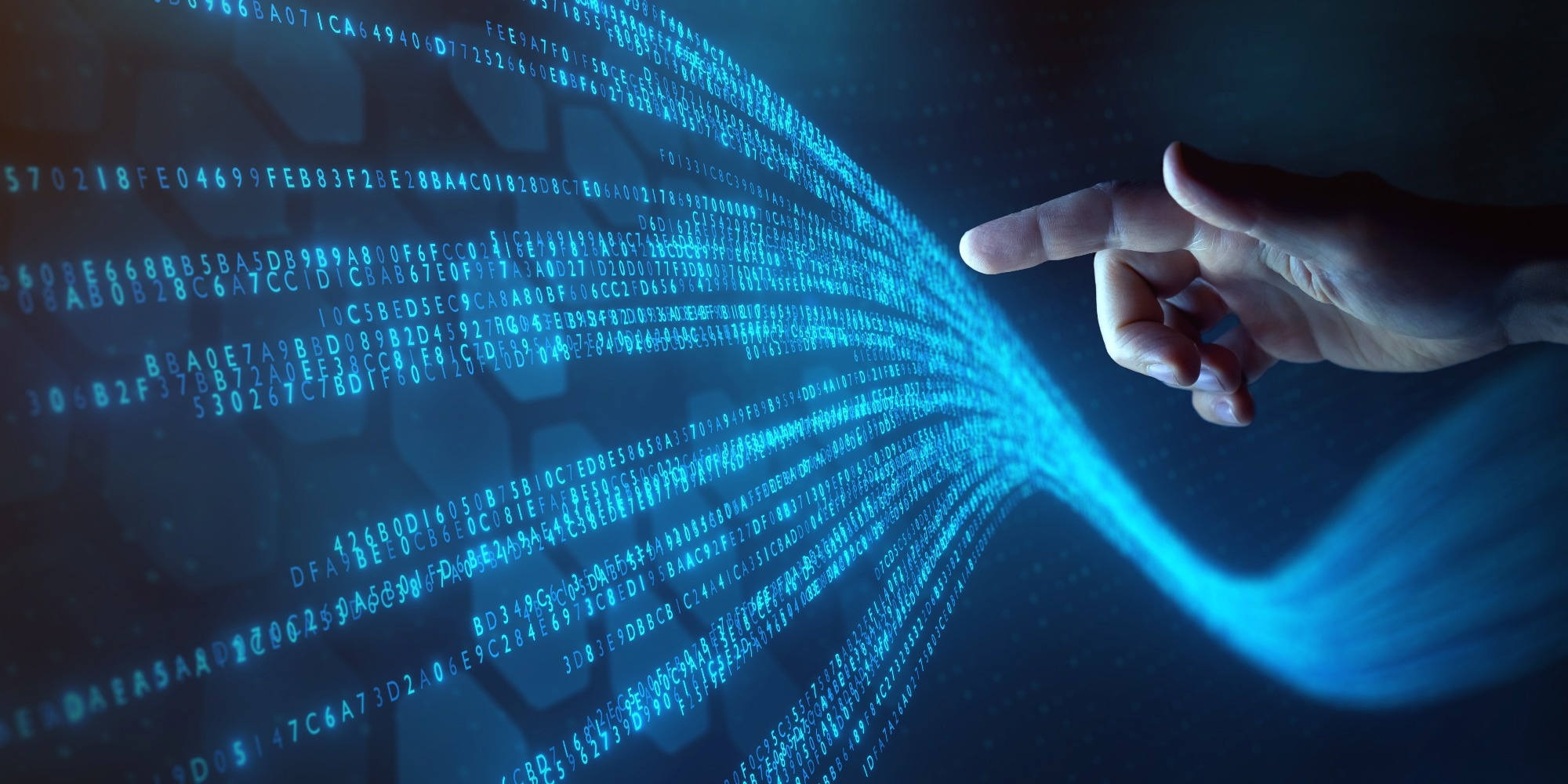
Image Credit: NicoElNino/Shutterstock.com
Do you think AI agents are especially helpful for less experienced users or for standardizing image acquisition in microscopy?
I believe that in the near future, AI agents will be capable of operating instruments at the level of a beginner or moderately trained user. They could also serve as valuable tools for training novice operators. In the long term, we may reach a point where pressing a button would allow the microscope to function with the performance level of a mid-tier operator.
I am not convinced that AI agents will soon match the skill level of highly qualified human experts. Humans tend to be exceptionally proficient in their tasks, and over the past 20 years, machine learning models have generally plateaued slightly above average human performance.
There are, of course, notable exceptions. For instance, AI algorithms for playing chess or Go can operate at a superhuman level. But this is primarily because the rules of those games are well-defined and unchanging.
AI still struggles to respond to the unknown, such as new environments, unfamiliar samples, or unexpected experimental conditions. That is one reason why fully autonomous vehicles remain a work in progress rather than a daily reality.
That said, AI performs remarkably well in domains where the rules are clearly defined and mature workflows exist, allowing for the collection of large volumes of training data. These are the environments where AI can truly excel. But when it comes to venturing into the unknown and doing something entirely new, which lies at the heart of scientific research, AI is not quite there yet.
For those who might want to begin automating experiments in their own labs, how would you suggest they start addressing that challenge?
I am not entirely convinced that an approach based solely on available data is the most effective path forward. There are two reasons for this skepticism.
First, if we consider how humans learn, it typically involves a very small amount of data. For example, compare the number of games a human chess master plays over a lifetime to the number of games AlphaZero or AlphaGo needed to reach human-level performance—the difference is several orders of magnitude.
Similarly, when learning to drive, we require relatively little training data before we can perform competently. The same applies to learning how to operate a microscope. The human brain is remarkably adaptable and capable of learning new tasks with far less data than what machine learning algorithms typically require.
So, whenever I hear a proposal that suggests building a machine learning system to operate a microscope entirely from scratch using a data-intensive approach, I have some doubts. In general, scientific research and microscopy do not naturally lend themselves to "big data" in the traditional sense.
The second aspect is that there are indeed scenarios where large datasets are appropriate and effective. This includes fields like medical imaging or industrial contexts such as the semiconductor industry, where workflows are mature and standardized. In those environments, big data plays a critical role.
In exploratory research, where conditions change frequently and new questions are constantly being asked, it remains a challenge. Human operators are still far better at navigating these fundamentally new situations, whereas machine learning has limitations in this area for the time being.
That said, the field is progressing very rapidly. We now have access to generally trained models such as SAM by Meta, which can be deployed straight out of the box. We have experimented with integrating SAM into imaging workflows for automated electron microscopy.
These models currently address only very specific tasks, such as image segmentation. While they can assist human operators, they are not yet capable of making the kinds of decisions that humans make during an experiment. So, while big data is helpful, it is not sufficient on its own.
Conversations on AFM #4: Automation of SPM with AI
Video Credit: Bruker BioAFM
What do you see as the main challenges to bringing automated experimentation into laboratories in the future?
When we first started working on these kinds of projects at Oak Ridge roughly 10 to 15 years ago, one of the biggest challenges was engineering control, whether we were using scanning probe microscopes or electron microscopes. You might have a machine learning agent written in Python or MATLAB, but at the end of the day, that agent had to send commands directly to the instrument—a step that was often difficult to implement.
Today, the situation is quite different. Many companies now offer APIs (application programming interfaces) that make it possible to control instruments directly through environments like Python.
A decade ago, that kind of support just didn’t exist. I remember in our early attempts at automating experiments with an electron microscope, one of the key concerns was the potential risk to the instrument’s service contract.
The instrument cost about $5 million, and if we connected it to a custom-built controller, there was concern over what that might mean for support or warranty. With scanning probe microscopes, this was somewhat easier to navigate due to their lower cost and risk. At the time, that was the primary limitation.
Now, many commercial manufacturers offer APIs or are planning to do so. In some cases, they are even willing to work with customers directly to provide certain functions. So, this aspect is improving.
The second key component is the machine learning algorithms. Fifteen years ago, those were much less accessible. It may be hard to imagine, but twenty years ago, we could not even run simple principal component analysis (PCA) on realistic datasets.
At the time, AFMs were generating more data than desktop computers could reasonably handle. Although the data output from AFMs hasn’t changed much—it's still limited by the physics of imaging—our ability to process that data has improved dramatically. Today, you can find nearly any algorithm you need on GitHub, and in many cases, these tools are already well-tested and ready to use.
One of the most challenging and often overlooked aspects of AI-driven experimentation is workflow design. When a human operates an instrument, they make a series of decisions in sequence. That sequence forms a workflow. You can think of it in different ways: as a step-by-step path toward a goal, as a search through a space of possible actions, or as an interaction with a constantly evolving environment—where new features or data points appear on the screen in real time.
At its core, workflow design is about replicating that human decision-making process using an AI agent. And that’s where things get complicated.
Many people underestimate this step. Here’s a simple way to frame the problem: imagine you have full access to the instrument and its API. It will do exactly what you tell it to do.
So, what should it do? That question is harder to answer than it seems. Even when using the same instrument and working with the same sample, different human operators often take entirely different approaches to their experiments.
Where do you see the future of real-time decision-making and data analysis, and what role does quantum computing play?
Human decision-making is shaped by prior knowledge, real-time data from experiments, and, crucially, hypothesis formation. Even decisions as personal as choosing a career path involve forming and testing a hypothesis. But there's another key factor that often sets the direction for everything: reward.
In essence, a reward can be almost anything. It might be becoming well-established in a field, discovering new physics, or understanding structure–property relationships in materials. From an AI perspective, though, we need to distinguish between rewards and objectives. Objectives drive us toward long-term goals; rewards are measurable outcomes that can emerge within a specific experiment.
Take, for example, an experiment where the goal is to tune a microscope to achieve a certain resolution; that resolution becomes the reward. Or consider a case where we aim to train a machine learning model that predicts local current-voltage (IV) curves or polarization hysteresis loops from local microstructure data. In that scenario, the model’s accuracy or predictive performance can be framed as the reward.
Here’s the critical point, one that, in my view, is absolutely central to automated and autonomous experimentation: AI is only useful if the reward function is clearly defined. Once a reward function exists, relatively simple optimization algorithms can be deployed to operate the microscope and attempt to maximize that reward within the allowed set of actions.
Humans are uniquely skilled at moving between objectives and rewards, thinking both forward and backward. Simple AI agents, especially those running scientific instruments, don’t have that flexibility. They rely on humans to define the reward function. With that in place, we don’t need complex AI systems; basic optimization workflows will do the job.
So, where do reasoning systems, large language models (LLMs), or more advanced ML agents come into play? Their value lies in helping humans articulate reward functions from higher-level objectives. This is where a top-down approach (using LLMs to clarify goals and design reward structures) complements a bottom-up approach (running optimization workflows on instruments). When the two align, the system becomes far more effective.
That said, setting long-term objectives remains a human responsibility. At some level, determining what we want to do isn’t just a scientific problem; it edges into philosophy and personal agency. It becomes a question of free will, and that’s well beyond the scope of AI.
About Prof. Dr. Sergei Kalinin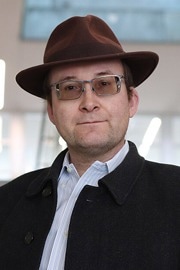
Prof. Dr. Sergei Kalinin is a leading scientist in the field of machine learning for physical sciences, with a Ph.D. in Materials Science from the University of Pennsylvania. He spent nearly two decades at Oak Ridge National Laboratory, advancing from Wigner Fellow to Corporate Fellow. His work focuses on data-driven discoveries in microscopy, atom-by-atom fabrication, and autonomous experimentation. Kalinin has held key roles at Amazon, Pacific Northwest National Laboratory, and the University of Tennessee. A recipient of major honors including the Feynman Prize and a Blavatnik Laureate, he is a Fellow of multiple scientific societies, a member of Academia Europaea, and a member of National Academy of Inventors.
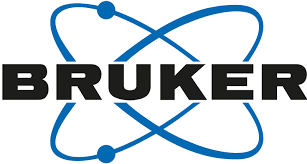
This information has been sourced, reviewed and adapted from materials provided by Bruker BioAFM.
For more information on this source, please visit Bruker BioAFM.
Disclaimer: The views expressed here are those of the interviewee and do not necessarily represent the views of AZoM.com Limited (T/A) AZoNetwork, the owner and operator of this website. This disclaimer forms part of the Terms and Conditions of use of this website.