Fabrication of materials using data-based techniques is being welcomed as a new strategy that will replace human scientists' hit and miss tests and labor demanding jobs. In an article posted to the Research Square / Nature Portfolio Journal* preprint server, a Robotic Scientist framework that may provide unparalleled capabilities for logical design, retrosynthesis, and programmable fabrication of nanoparticles is discussed.
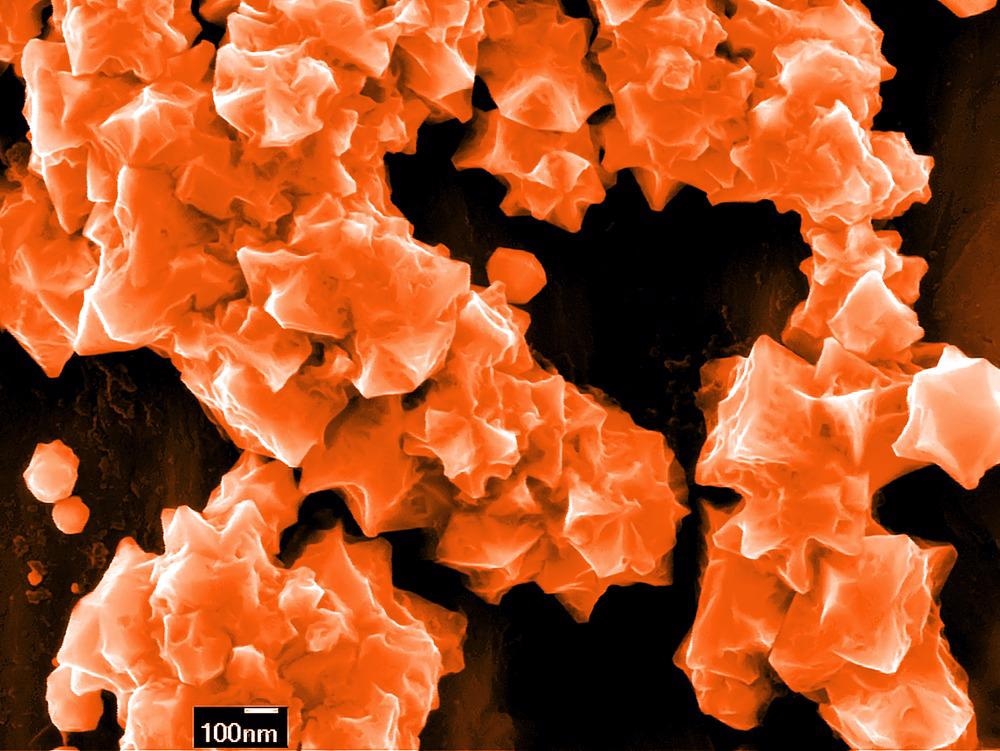
Study: Towards a Robotic Scientist for Synthesis of Nanocrystals. Image Credit: Georgy Shafeev/Shutterstock.com
The Robotic Scientist framework is taught to fabricate gold nanocrystals by using multidisciplinary domains such as artificial intelligence, automated robotics, and big data.
Data-Driven Fabrication of Materials
Data-guided development of materials is being hailed as a new paradigm for shifting laborious activities and trial-and-error tests away from human researchers and towards robotic scientists or chemical fabrication mechanized systems.
The sophisticated Human-AI-Robot cooperation system is expediting the multidisciplinary breakthrough in the fabrication of materials towards a Robotic Scientist for mechanized creation.
Convergence of chemical research, theoretical modeling, purpose-driven databases, configurable cyber networks, and mechanized physical systems is required in this growing discipline.
One of the potential objectives is digitized material generation, which involves gradually collecting information, efficiently revealing data links, and producing viable solutions over time based on prior iterations.
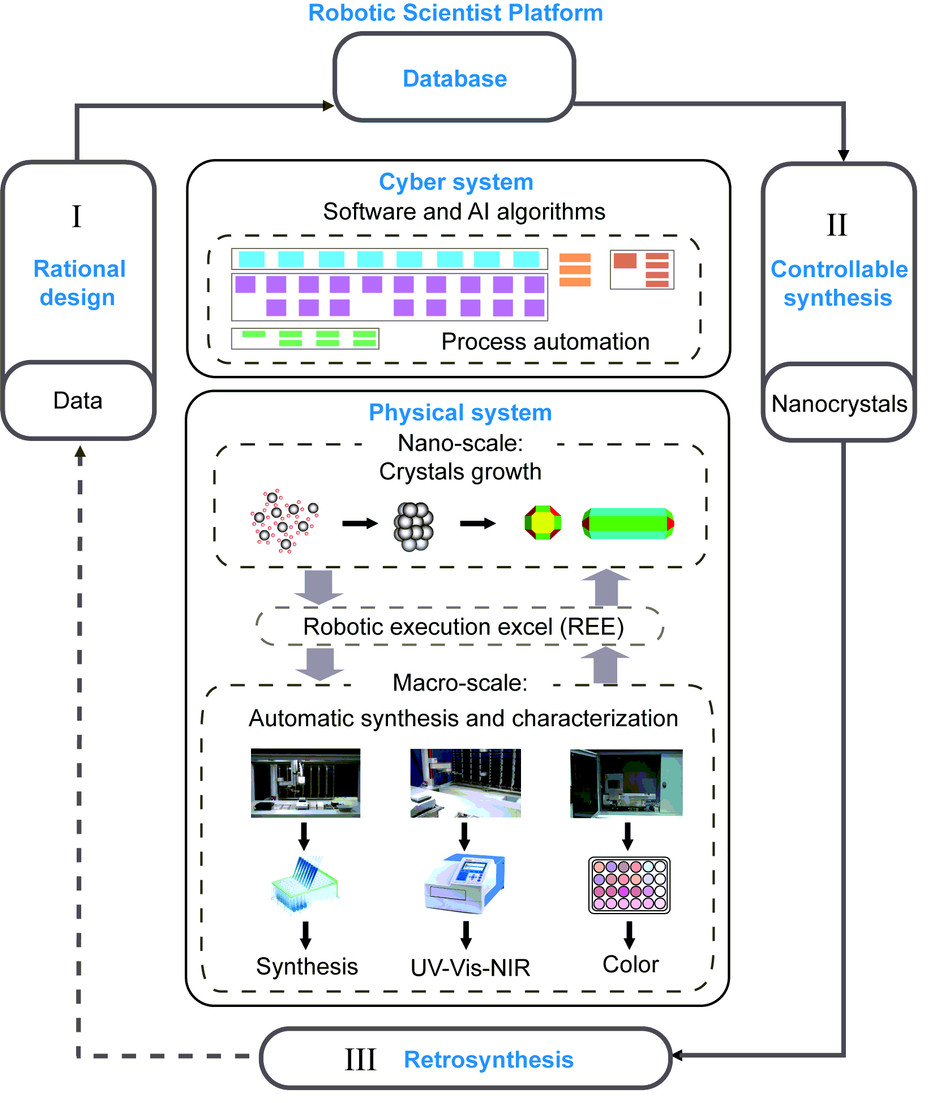
Figure 1. Robotic Scientist platform. Convergence of the database, cyber system, and physical system and process flow: I. Rational design, II. Controllable synthesis, and III. Retrosynthesis for closed-loop synthesis of nanocrystals based on the Robotic Scientist platform. © Zhao, H., Chen, W., et al. (2022)
Existing Work on Automated Fabrication Processes
Significant endeavors have been undertaken in the last decade to achieve digital production of substances.
On the macro-scale, layer-by-layer computerized additive production of 3D substances has been established. Artificial biology is a micro-scale milestone for the computerized fabrication of biomaterials using cells as the hardware on which genes are programmed
Lately, there has been considerable growth in biological programming languages and autonomous systems for chemical synthesis on a small scale. Simultaneously, a computerized chemist has been reported in order to find photocatalysts, opening the door to automated synthetic material research on the micro-scale.
Nonetheless, there are several limits to computerized fabrication, such as material searches lacking conceptual models, blind modification of substances without science-based methodology, and a lack of hardware-software integration to enable material innovations.
As an example, this study illustrates how the Robotic Scientist framework, which allows logical design, controlled fabrication, and retrosynthesis of nanocrystals, may address these challenges.

Figure 2. Illustration of the Robotic Scientist platform. a, Photograph. b, Schematic representation. The color frames in the photograph and schematic representation match each other. Backrest: Storage for the sample, microplates and pipette tips; Central line: Mobile robot for microplate transport; Top: Three automatic pipettors for liquid handling; Bottom: Mobile color-ultra-sensitive camera for in situ color characterization; Platform: Synthesis platform for in situ sampling; Instrument: Microplate reader for in situ UV-Vis-NIR absorption spectrophometry; Right circle: Robotic arm for instrument services. © Zhao, H., Chen, W., et al. (2022)
Advantages of the Proposed Robotic Scientist
Educating scientists with the necessary expertise requires significant resources, and alternative biochemical and material synthesizing processes might result in a wide range of results, even for qualified professionals.
Furthermore, the majority of artificial synthesis is trial-and-error and arduous, with inevitable inadvertent errors.
The Robotic Scientist framework reported is a significant development in automation relevant to nanocrystal production and represents an important leap towards data-guided materials development.
The merging of Robotic Scientist-aided production on the macro level and nanocrystal development on the nanoscale results in a complex tight loop comprising logical design, controlled fabrication, and retrosynthesis.
Here, existing chemical information based on data analytics, thermodynamics and kinetic models, and machine learning models were coupled to speed logical design of nanocrystal structure given initial assumptions.
To prevent unguided tuning of materials, orthogonal tests, as well as one, two, and three-factor experimentations, were carried out in cycles, and a database was built for successful training of the machine learning models to allow controlling the fabrication of nanoscale crystals.
The readily available large data set (on-site categorized UV-Vis-NIR absorption spectra and RGB color results) and smaller data set (ex-situ TEM validation) were produced in these procedures to ascertain the Au nanocrystals genome, and genome understanding plays a critical role in assisting the retrosynthesis operation.
The researchers proved that the Robotic Scientist can be taught in the same way as a human scientist can for retrosynthesis and scalable fabrication of the desired gold nanocrystals.
Using the Robotic Scientist platform, this effort centers on developing a closed-loop (design-synthesis-retrosynthesis) of automation in nanoscale crystal fabrication.
Even though a full Robotic Scientist was an idealistic goal, the developed model is a solid stepping stone toward a Robotic Scientist with the key abilities of scientific hypotheses, tests by combining hardware and computer components, and result interpretation.
Future initiatives are expected to narrow the gap, with ultimate automation of all phases of nanocrystal production.
Although the Robotic Scientist was only shown for gold nanocrystals in this study, the findings show that automation has the potential to expedite data-driven materials discovery on the nanoscale.
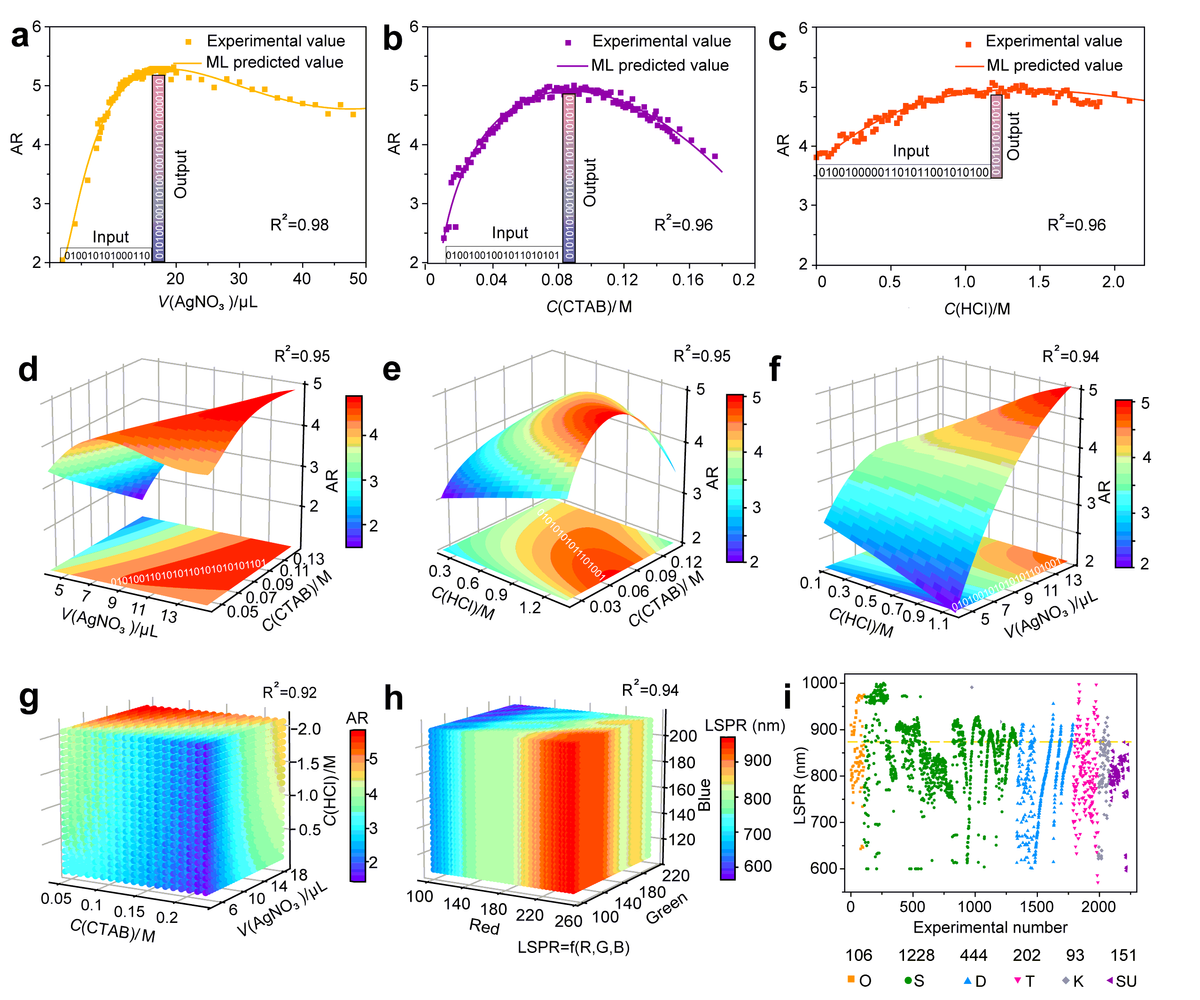
Figure 3. Controllable synthesis, ML prediction, and database construction. a-c, Single-factor ML predicted models. d-f, Double-factor ML predicted models. g, Triple-factor ML predicted models. h, LSPR-color model. i, Overview of the number of experiments: O, S, D, T, K, and SU represent the orthogonal, single-, double-, triple-factor, kinetics, and scale-up experiments, respectively. The relationship between the experimental factors (as inputs) and AR (as outputs) is identified, and ‘01010101’ is the schematic diagram of the controllable range. © Zhao, H., Chen, W., et al. (2022)
*Important Notice
Research Square / Nature Portfolio Journal publishes preliminary scientific reports that are not peer-reviewed and, therefore, should not be regarded as conclusive or treated as established information.
Reference
Zhao, H., Chen, W., et al. (2022). Towards a Robotic Scientist for Synthesis of Nanocrystals. Available at: https://www.researchsquare.com/article/rs-1219605/v1
Disclaimer: The views expressed here are those of the author expressed in their private capacity and do not necessarily represent the views of AZoM.com Limited T/A AZoNetwork the owner and operator of this website. This disclaimer forms part of the Terms and conditions of use of this website.