With the use of integration of high-powered X-Rays, machine learning, and phase-retrieval algorithms, Cornell scientists showed the intricate nanotextures in thin-film materials, providing researchers with a novel, streamlined method to examining likely candidates for microelectronics and quantum computing, among other applications.
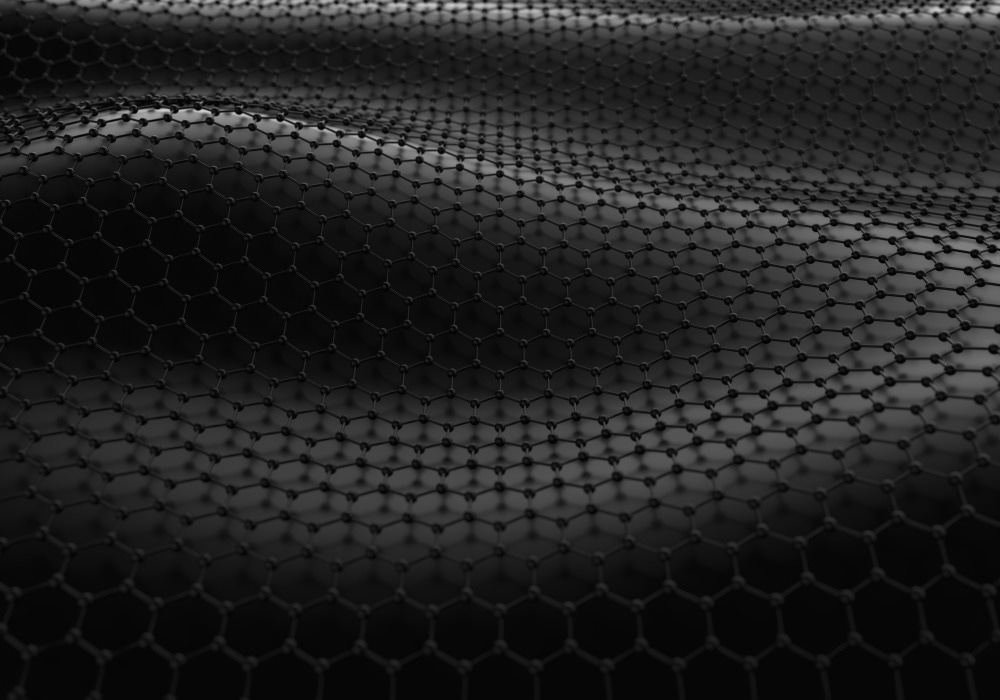
Image Credit: videoduck/Shutterstock.com
Researchers are specifically keen on nanotextures that are dispersed unevenly across a thin film as they can provide the material with novel properties. The most efficient way to study the nanotextures is to visualize them, a challenge that usually needs complex electron microscopy and does not preserve the sample directly.
The novel imaging technique explained on July 6th, 2023, in the Proceedings of the National Academy of Sciences wins over these difficulties with the use of machine learning and phase retrieval to invert traditionally gathered X-Ray diffraction data—like the one generated at Cornell High Energy Synchrotron Source, where information for the research was gathered—into real-space visualization of the material at the nanoscale.
The application of X-Ray diffraction makes the technique more available to researchers and enables for imaging a greater portion of the sample, stated Andrej Singer, Assistant Professor of Materials Science and Engineering and David Croll Sesquicentennial Faculty Fellow in Cornell Engineering, who headed the study with doctoral student Ziming Shao.
“Imaging a large area is important because it represents the true state of the material,” Singer stated. “The nanotexture measured by a local probe could depend on the choice of the probed spot.”
Another benefit of the novel approach is that it does not need the sample to be broken apart, facilitating the dynamic research of thin films, like presenting light to observe how structures emerge.
“This method can be readily applied to study dynamics in-situ or operando,” Shao adds. “For example, we plan to use the method to study how the structure changes within picoseconds after excitation with short laser pulses, which might enable new concepts for future terahertz technologies.”
The technique was assessed on two thin films, the first of which had a recognized nanotexture employed to confirm the imaging outcomes. After testing a second thin film—a Mott insulator with physics linked to superconductivity—the scientists found a new kind of morphology that had not been noticed in the material earlier—a strain-induced nanopattern that creates at the same time during cooling to cryogenic temperatures.
“The images are extracted without prior knowledge, potentially setting new benchmarks and informing novel physical hypotheses in phase-field modeling, molecular dynamics simulations, and quantum mechanical calculations,” Shao adds.
Co-authors comprise the late Lena Kourkoutis, Associate Professor of Applied And Engineering Physics; Kyle Shen, the James A. Weeks Professor of Physical Sciences in the College of Arts and Sciences; Darrell Schlom, the Herbert Fisk Johnson Professor of Industrial Chemistry and Tisch University Professor in the Department of Materials Science and Engineering; and Hari Nair, Assistant Research Professor of Materials Science and Engineering.
The study was financially supported by the US Department of Energy and the National Science Foundation.
Syl Kacapyr is Associate Director of Marketing and Communications for Cornell Engineering.
Journal Reference:
Shao, Z., et al. (2023) Real-space imaging of periodic nanotextures in thin films via phasing of diffraction data. Proceedings of the National Academy of Sciences. doi.org/10.1073/pnas.2303312120.