Despite the progress in technology and exploitation of automatic speech recognition, technological barriers to flexible solutions and user satisfaction still exist under certain circumstances. These barriers are related to several factors, such as sensitivity to the environment (background noise), speech disorders, and limited privacy.
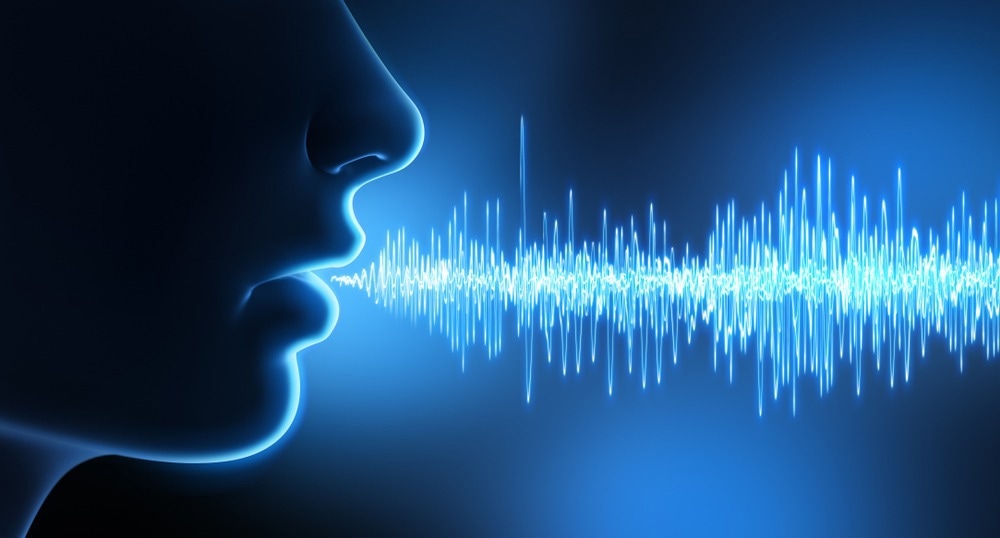
Study: Bioinspired dual-channel speech recognition using graphene-based electromyographic and mechanical sensors. Image Credit: peterschreiber.media/Shutterstock.com
In a study published in Cell Reports Physical Science, a dual-channel graphene-based electromyographic and mechanical sensor was proposed for the simultaneous collection of two bio-signals for speech recognition applications.
Electromyographic graphene electrodes developed in the present study showed better performance than commercial electrodes. Moreover, during 10 million fatigue tests, the graphene-based mechanical sensors (or graphene sensors) showed excellent repeatability.
The combined performance of electromyographic graphene electrodes and graphene sensors resulted in 96.85% accuracy on a 71-word dataset and 100% on the digit dataset. Additionally, the graphene sensors developed in this study showed excellent resistance to noise with >95% accuracy. Thus, the present study confirms the efficiency of dual biological channels in improving speech recognition performance.
Human-Computer Interactions with Speech-Recognition System
Human-computer interaction is a primary issue in the information technology era. Hence, designing a convenient and user-friendly interface has become a critical technological issue. Machine-dependent interfaces limit the use of computers in most populations. Speech enables a suitable form of communication for human-machine interaction.
Presently, many human-machine interface systems have made extensive use of verbal voice recognition. Siri and Cortana, among other voice assistants, have made this possible because of the advancements in automated speech recognition technology.
However, several unique situations make it difficult to use automated speech recognition systems. For example, sound transmissions are severely hampered by external noise in loud environments or uncomfortable circumstances, such as private discussions or speech issues, and sound signals can be too faint to be heard.
A speech-recognition system based on sound and other relevant information is essential to broaden its possible applications. The signals of electrocorticography (ECoG), electromyography, electroencephalography (EEG), and articulatory activity have been used in speech recognition.
Acquiring a high identification rate for EEG data is challenging because of its low signal-to-noise ratio (SNR). While acquiring higher identification is possible with ECoG signals, this technique requires intracranial insertion of electrodes. Silent speech recognition based on electromyographic signals can achieve greater accuracy. However, better accuracy necessitates the installation of eight electrodes on the human skin, thereby substantially reducing its wearability.
(A) Schematic showing the mechanism of the dual biological channels. (B) DGEMS with key components labeled. Each patch contains two DGEMS. Two patches are placed on the chin and throat to collect bio-signals simultaneously, and the corresponding waveforms are shown in the bottom graph. (C) Photographic image of a DGEMS. Scale bar, 1.5 cm. (D) Schematic of the frequency spectrum. The yellow line represents EMG signals, and the red line represents mechanical signals. (E) Working principle of speech recognition. The features of EMG and mechanical signals are extracted through the neural network and fused. Then, the fused features are used for classification. © Tian, H et al. (2022).
Dual-Channel Speech Recognition
Previously, a speech generation chain was used for silent speech recognition based on a single signal and contained minimal speech-related information. This speech recognition process required a multielectrode configuration.
Thus, researchers have analyzed the speech generation mechanism and utilized a dual biological channel-based speech recognition system to realize high accuracy with a limited number of sensors and address the drawbacks of conventional single-type biological signals.
Previous analysis revealed that an action potential is produced in the motor cortex based on the expression generated in the brain. This action potential is later transmitted to muscle fibers through neurons, thereby inducing contraction. In addition, the ion channels of the muscle open, releasing potassium (K+), sodium (Na+), and calcium (Ca2+) ions.
Surface electrophysiology electrodes may transform these ion currents into electron currents, which are then employed as electromyographic signals to represent the strength of the muscle contraction. Consequently, the surface of the skin could be distorted by muscular contraction.
However, researchers anticipated that the mechanical properties of the dermis, epidermis, and subcutaneous tissue and the link between muscles and bones could impact the skin deformation that could be transmitted. Hence, mechanical graphene sensors can be used to detect skin surface deformation.
In the present work, the physiological signals of the speech generation chain, which can be easily connected and have a high SNR, were combined with a graphene sensor based on a dual biological channel that could concurrently record electromyographic and mechanical signals.
The two biological signals transmitted distinct speech-related data and combining the two signals improved the speech recognition accuracy while using fewer electrodes and making the device more wearable.
Furthermore, the chin and throat have several overlapping muscles; therefore, the acquired electromyographic signals usually include action potentials from various muscle fibers. Thus, the underlying speech-related elements were analyzed in the signal rather than trying to determine the source of muscle movements.
Conclusion
Overall, dual biological channel-based speech recognition and dual-channel graphene-based electromyographic and mechanical sensors to simultaneously collect two bio-signals were presented in this study.
The graphene electrodes in the present study had lower electrode-skin impedance than the commercial electrodes. In contrast, graphene sensors showed excellent repeatability even after 10 million cycles of testing.
While electromyographic signals had more information at low frequencies, mechanical signals had more information at high frequencies. Moreover, the 71-word and digit datasets achieved 96.85% and 100%, respectively.
A potential method for enhancing speech recognition performance in complex environments was introduced in this study. Furthermore, integrating a near-sensing computing architecture with dual-channel graphene-based electromyographic and mechanical sensors can accomplish intelligent sensory processing.
Reference
Tian, H et al. (2022). Bioinspired dual-channel speech recognition using graphene-based electromyographic and mechanical sensors. Cell Reports Physical Science. https://doi.org/10.1016/j.xcrp.2022.101075
Disclaimer: The views expressed here are those of the author expressed in their private capacity and do not necessarily represent the views of AZoM.com Limited T/A AZoNetwork the owner and operator of this website. This disclaimer forms part of the Terms and conditions of use of this website.