In the last five years, the discovery and significant interest in twisted bilayer graphene gave rise to an entirely new subfield in advanced materials science and quantum physics: twistronics. In a new study, scientists apply machine learning algorithms to discover more about this remarkable phenomenon.
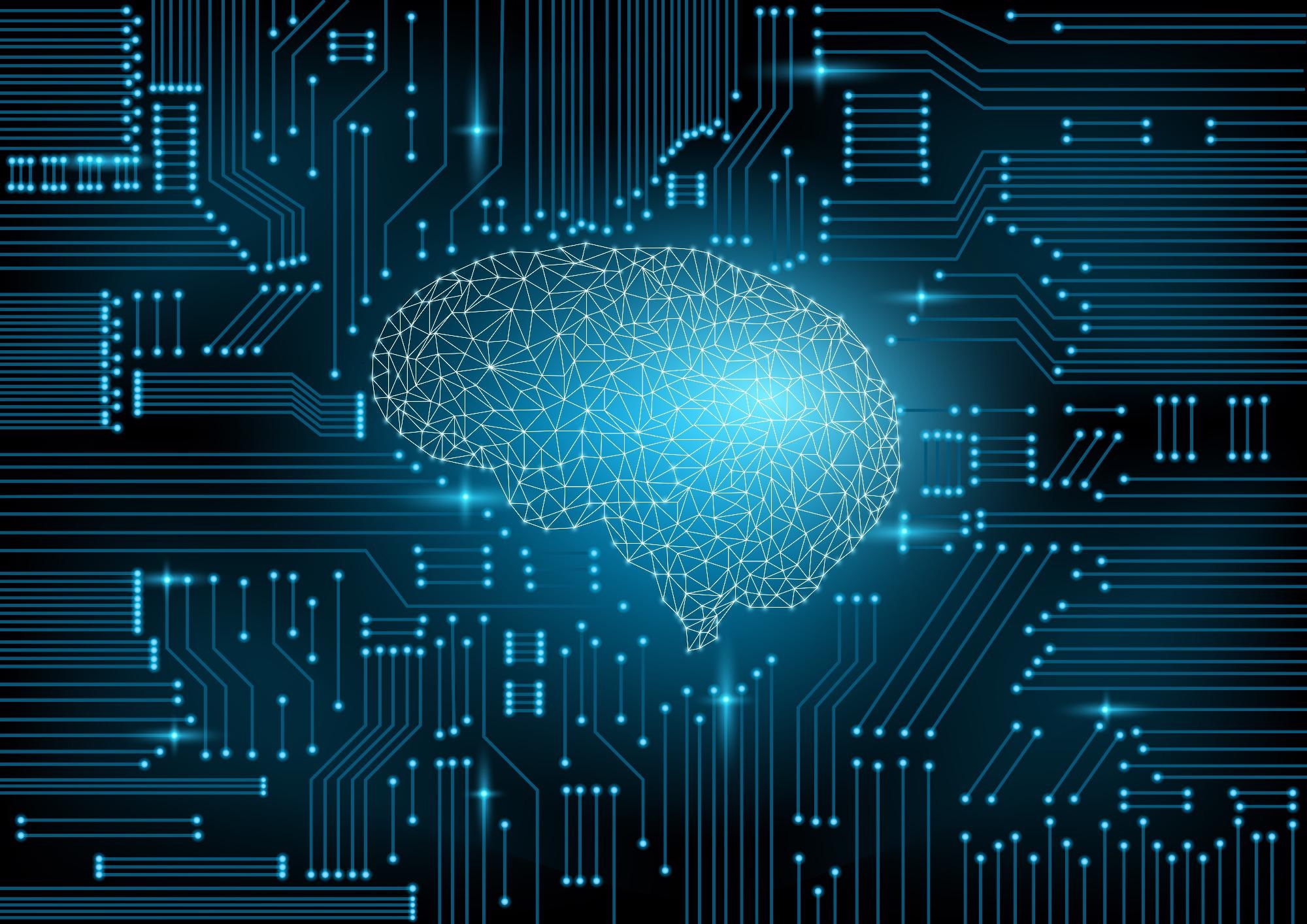
Image Credit: CNStock/Shutterstock.com
Discovering the Magic Angle: Twisted Bilayer Graphene
The discovery of two-dimensional graphene rocked the scientific world when it was published a few decades ago. Since then, graphene innovation has advanced significantly in terms of synthesis methods, understanding of its unique properties, and development of practical applications.
In 2018, researchers working at the US Massachusetts Institute of Technology (MIT) discovered that they could make graphene superconductive by stacking two atom-thick layers of the material on top of each other at a precise 1.1 ° angle.
At this orientation, which the researchers referred to as the “magic twist angle,” bilayer graphene transforms from a weakly correlated Fermi liquid into a strongly correlated two-dimensional electron system. The properties of the system are exceptionally sensitive to carrier density, the proximity of nearby gates, and variation in the twist angle.
Twisted bilayer graphene was found to have several unique properties. It is superconductive, but it also has interaction-induced insulating states, electronic nematicity, linear in temperature low-temperature resistivity, quantized anomalous Hall states, and magnetism.
Challenges in Twistronics
The discovery of twisted graphene gave rise to a new subfield in materials science and quantum physics: twistronics.
Scientists working in this field are now looking for more two-dimensional materials and stacking angles that could produce remarkable properties.
Researchers are focused on finding magic angles for other van der Waals materials (like graphene) that exhibit a magnetic ground state – be that antiferromagnetic or ferromagnetic – when they are produced in ultra-thin layers.
But discovering magic angles like graphene’s 1.1 ° angle is no simple task.
High-resolution microscopy with techniques like transmission electron microscopy (TEM) or scanning probe microscopy (SPM) can help researchers precisely measure the angles of twist to below 0.01 ° accuracy. These techniques, however, are time-consuming and require samples to be either free-standing or supported on a conductive substrate.
Not only this, but these measurements can also only provide very local information about areas smaller than a micrometer – twist angles can vary considerably within just a few micrometers.
Practically, then, this approach is not viable. Practical applications for twisted bilayer materials require characterizations on arbitrary substrates over a much larger area and in a relatively shorter time frame.
Other techniques include transport measurements of devices under magnetic fields and at low temperatures, but this approach is complex and limited to small areas.
Low energy electron diffraction (LEED) provides information about the layers stacked together and their orientations, covering larger areas than TEM or SPM. However, the method also requires a conductive substrate and high vacuum conditions.
Raman spectroscopy is a promising technique for bulk identification of magic angles because it obtains a large amount of information about the material’s state from its Raman spectrum at once. However, Raman spectra differences are often extremely subtle, and so using the technique to manually identify twist angles is prohibitively time-consuming and tedious.
Using Machine Learning to Advance Graphene Innovation
Quick, accurate, nondestructive methods for discovering twist angles are needed to continue innovation in this field.
In new research, scientists at Kyushu University, Japan, propose a machine learning analysis technique to automatically classify the Raman spectra of twisted graphene samples into a range of twist angles.
The study was published in the journal Applied Nano Materials in 2022 alongside an open-source codebase for the machine learning algorithm that researchers developed.
This algorithm has low computational demands; it is fast and has around 99% accuracy compared with manual spectra labeling.
The method described benefits from the flexible, non-invasive nature of Raman spectroscopy measurements combined with the speed and predictive accuracy of machine learning. As a result, the authors argued that it could facilitate exploration in the emerging field of twistronics.
The method involves extracting features of the Raman spectrum of twisted graphene, which were used to train the machine learning model to infer the twist angle within predefined ranges.
The flexibility of the Raman spectroscopy technique means that the method can also be expanded to determine how much strain and doping are present in graphene samples.
The method could also, said researchers, be applied to almost any other heterostructure. This means that the field of twistronics can use it to find more materials and magic angles which may well see significant practical applications in the next few years.
Not only this, but the research also demonstrated how open source machine learning algorithms could enable the easy and effective integration of artificial intelligence into other research techniques and fields.
References and Further Reading
Andrei, E.Y., and A.H. MacDonald (2020). Graphene bilayers with a twist. Nature. doi.org/10.1038/s41563-020-00840-0.
Cao, Y., V. Fatemi, S. Fang, et al. (2018). Unconventional superconductivity in magic-angle graphene superlattices. Nature. doi.org/10.1038/nature26160.
Park, J-G (2016). Opportunities and challenges of 2D magnetic van der Waals materials: magnetic graphene? Journal of Physics: Condensed Matter. doi.org/10.1088/0953-8984/28/30/301001.
Pilkington, B. (2022). What is Twisted Graphene? [Online] AZO Nano. Available at: https://www.azonano.com/article.aspx?ArticleID=5998
Solís-Fernández, P., and H. Ago (2022). Machine Learning Determination of the Twist Angle of Bilayer Graphene by Raman Spectroscopy: Implications for van der Waals Heterostructures. Applied Nanomaterials. doi.org/10.1021/acsanm.1c03928.
Disclaimer: The views expressed here are those of the author expressed in their private capacity and do not necessarily represent the views of AZoM.com Limited T/A AZoNetwork the owner and operator of this website. This disclaimer forms part of the Terms and conditions of use of this website.